Following up on my last blog post on a new framework for systemic change, I would like to present here the main methodology we used to measure whether there have been transformations in the attitudes of farmers. The approach we used was Cognitive Edge’s SenseMaker®, which allowed us to deeply scan for changes in attitudes and beliefs beyond mere observation of changed behaviours.
The SenseMaker data collection process has three steps:
- By asking for a specific experience, the respondents’ memory is triggered to mentally place them in a situation related to the field of interest that they have lived through.
- Respondents record a narrative of that experience and, through so doing, bring the experience into working memory.
- Respondents are asked to signify (interpret) their own narrative using a set of carefully crafted questions.
‘Self-signification’ of the stories by the respondents is a key aspect of this method. It allows respondents to interpret their own experiences, avoiding expert bias. Self-signification furthermore enables respondents to give additional meaning to each situation that is not explicitly expressed in the story. This provides a rich set of quantitative meta-data for analysis.
For this study, respondents were asked to share a specific experience of support they have received from people outside their family related to farming. They were explicitly invited to share both positive and negative experiences. After sharing an experience, which was recorded with a mobile device, the respondents were asked to answer a number of signification questions built up of triads (Figure 1), dyads (Figure 2) and multiple-choice questions.
Respondents are asked to place a dot in the triad to the left in Figure 1, signifying how the story represents the blend of the three aspects in the corners. All the dots of all the stories are then visualised during the analysis as seen in the triad on the right of Figure 1. Colour coding allows differentiating between different characteristics of the respondent (e.g. gender, age bracket, poverty level, etc.). Individual stories remain accessible throughout the analysis to give context to the visualised patterns.

Figure 1 Respondents are asked to place a dot in the triad to the left, signifying how the story represents the blend of the three aspects in the corners. All the dots of all the stories are then visualised during the analysis as seen in the triad on the right.
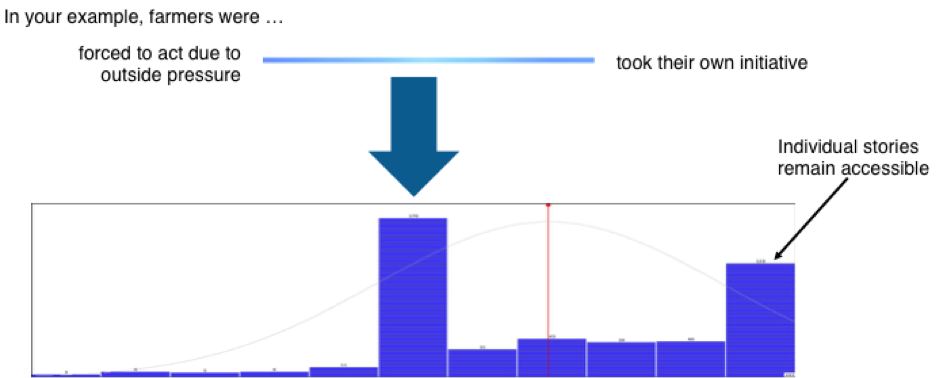
Figure 2 Respondents are asked to drag a ball between the two polarities of the dyad (above), which in this example represent pro-activeness and reactiveness of the farmers, respectively. All stories together can then be displayed as a histogram for the analysis.
In total, 497 stories were collected from four geographical areas. The characteristics of the sample are shown in Figure 3.
The sample was designed to be large enough for statistical analysis. It was also intended to assess the scale of observed changes. The sample population was divided into three groups: an intervention group, which was about 50% of the sample; a spillover group of farmers from adjacent areas where there is a potential for them to have profited from the interventions indirectly; and a comparison group from areas farther away without any contact to the intervention. The respondents were also asked to tell whether the support they mention in their story has always been available or has only become available in the last year – which is essentially the period in which interventions have been going on. As there is no baseline, this allows for a crude before/after comparison, in addition to the comparison between intervention, spillover and comparison groups.
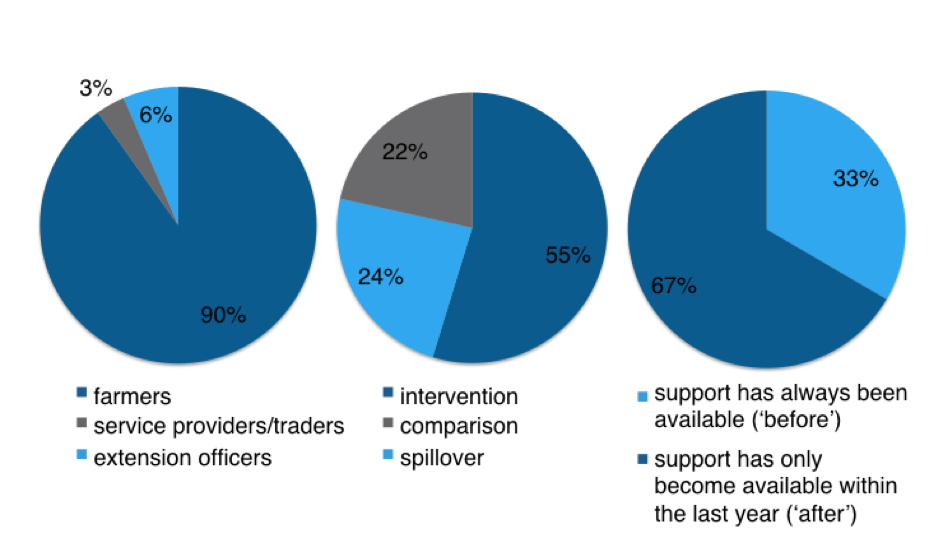
Figure 3 The sample of collected stories mainly comprised stories from farmers as they are the main beneficiaries (left). The sample was split into an intervention group, a spillover group and a comparison group (centre). As a crude before/after measure, the respondents were asked if the support their story was about has always been available or has only become available within the last year – the period in which interventions have been going on (right).
The analysis of the data was done in a collaborative effort between the two consultants involved and the project team.
The whole report of the study has now been published and can be downloaded here: Jenal 2016 – A New Framework for Assessing Systemic Change
I have followed Sensemaker for many years, as an outsider. I also use the concept of “self signification” in the context of systematically monitoring indicators of change in sets of published sources. One of the aspects of the process which we emphasise is the importance of using neutral language in our classification systems, avoiding loaded terms at all costs. In the triad which you cite, the terms Tradition and Nature are fine, but we would never use a term like Progress which comes with so much positive and (increasingly) negative baggage. Indeed, the same would apply to the term ‘development’. For your purposes, for example, how can you know whether respondents support or abhor Progress? I would have used Change as the term instead. I am not criticizing Sensemaker. I have always liked the methodology. I suppose I am arguing for standards of neutrality in sense-making application languages. I work hard to make our Inquiry Languages as disinterestedly neutral as possible. Although it is not perfect, the Inquiry Language behind our Environmental Change Monitor database is, I think, my best effort so far, especially given the emotionally charged nature of the subject domain. If you (and even Dave Snowden) would like to have a look at it, I would love to show you — as well as illustrate how our collections become self-signifying.
Hi Jan. Thanks for your comment. Generating signifiers is a challenging task and I couldn’t say I have reached perfection (if that is even possible). So, thankful for the thought about neutrality of signifiers. I will take this into account.
You can get an indication of whether people welcome or ‘abhore’ progress for example if you also collect the (self-signified) emotional tone of the story or when you read the actual stories. But it’s a fair point that it might not be obvious. But then again neutral signifiers can often be interpreted both ways – which is often an intended ambiguity.